How to build a successful AI Project – before it even begins
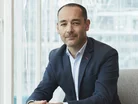
The expansive role of AI in business is now inevitable, rather than merely desirable. But executing a successful AI project can be a towering task – and one that doesn’t always work.
Statistics online suggest some 70% of AI projects fail, though according to a global IDC survey, just over a quarter (28%) of businesses reported AI failure in 2021.
This variation in figures on AI project failure demonstrates the importance of implementing a robust planning strategy.
And according to Gregory Herbert, SVP and GM, EMEA at Dataiku, this starts with ensuring the fundamentals are in place before any given project is even off the ground.
One of these key fundamentals is actually choosing the right project, Gregory tells Business Chief.
But how to do that?
“It’s a case of tackling the time-honoured framework of 5Ws and 1H — but in a tailored order,” he says.
Who will this project benefit?
Gregory points to the success or failure of a data or AI project as being directly linked to who the target audience is. And so even before attempting to build the solution, companies must define the project’s specific audience – which can be either external or within the company itself.
“With this decided, organisations can then design the solution with the target audience’s application of it at the front of their mind. There’s nothing worse than having a project that goes unused and wastes data teams’ valuable time due to a lack of audience specificity.”
To avoid this, companies need the knowledge to both choose and understand the audience, explains Gregory, and this comes from collaboration.
“Data and AI project developers must work closely with business stakeholders and subject matter experts to define this audience – who is the audience? What are their needs? What are their current processes and habits?”
How will it improve experience or outcomes?
Once a company has honed-in on their audience, it’s time to measure what the return on investment (ROI) of the AI system will be. For this, it is necessary to ask – how will this AI use case be a money maker, a money saver, or open up a new capability for the target audience?
According to Gregory, if you can’t formulate a clear answer to this question, then it’s worth going back to the drawing board. “AI use cases should focus on opportunities that have tangible and measurable business results.
“Failing to ask the right ‘how’ questions from the outset to quantify AI use cases can put future projects at risk and inhibit garnering company-wide backing for AI initiatives. Teams must be able to present quantifiable benefits: These could include time saved, increased revenue, and risk avoidance.”
Why is using AI for this purpose better than the existing process?
Having worked out the project’s estimated potential value, before going any further, Gregory suggests asking if these anticipated benefits are worth the time and investment that will be needed to replace existing processes.
“If you can’t see any major improvement to ROI or value from using AI over current processes, then move onto the next use case. Don’t opt for a project just because you want AI to do it. Instead, put your energy into heavy-hitting applications that can offer value at scale or can create a new capability previously not possible.”
What is the upside if the project succeeds, and the the consequences if it fails?
At this stage, it becomes a case of honestly evaluating what will happen if the project succeeds and, in particular, if it potentially fails.
Gregory points to considerations in assessing such criteria as including the scope of the project, access to subject matter experts, and the availability of analytical resources, from both a human and infrastructure perspective.
He points to three questions, which can be particularly useful in answering the ‘what.’
- Will there be significant damage to brand reputation or customer trust?
- Will there be damage to the level of organizational trust in data and AI projects and the ability of data teams to execute?
- Are there risks regarding regulatory compliance or customer privacy?
Where does the data come from and is it appropriate for the objective?
Without data, there is no AI system, explains Gregory. The fact is, the majority of AI solutions utilise supervised machine learning algorithms that use a set of labelled data from which data scientists train the machine. The computer then learns from whatever examples it is fed. To work effectively and achieve highly accurate results, a vast amount of data is needed.
“If companies don’t have data readily available for the specific use case, they should, firstly, reduce any external dependencies for their first AI project. Organisational buy-in is crucial, and this streamlining reduces complexity and guides teams towards initial projects that have high speed to value potential.
“Secondly, this buy-in emerges from involving all the relevant stakeholders early and then keeping them in the loop while the project is being designed. This will drive collaboration across business, IT, and data science groups.”
When does a prototype need to be completed?
With all the previous questions asked and answered, it then becomes necessary to time it right.
Gregory offers up an analogy for this. Rather than trying to open a shop with all its products and services in one go, it’s better to offer a limited selection of items that can then be progressed and developed in line with how they are received.
“This is adopting the ‘progress before perfection’ and Minimal Viable Product mindset – which has a dual purpose of generating excitement and credibility with internal stakeholders about the vision of the project while also creating space for feedback and amendments.
“The best way to see how a model operates is in production, and so this methodology dramatically increases the chances of avoiding project failure.”
Project timelines should be formed from the outset, outlining the development to prototype to business operationalisation journey. With robust MLOps, Gregory says companies can easily make the transition from the exploratory phase to the actual deployment of the product.
“As outlined from the start, a fail-safe way of making sure a project makes its way through to production is opting for the right use case. In making that decision, it’s essential to lock down the fundamentals.
“By answering these six questions before embarking on your next — or first — data and AI project, you can bypass false starts and create a system that is geared for success.”
- Mira Murati: 10 things to know about the new CEO of OpenAILeadership & Strategy
- COP28 Talks: Microsoft Sustainability chief Sherif TawfikSustainability
- AI is changing the way we work and leaders must adapt – fastDigital Strategy
- Five things we learned at world’s biggest tech show GITEXTechnology
Featured Articles
SAP has announced it has appointed a new President for a newly-created EMEA region, aiming to make the most of the opportunities of cloud and AI technology
From fighter pilot to fashion house financier, Mohammed Alardhi has taken Investcorp to great heights – so what’s the secret to his success?
Dr Omar Al-Attas, Head of Environmental Protection and Regeneration at Red Sea Global, shares his COP28 hopes and approach to regenerative tourism strategy